Παρουσίαση/Προβολή
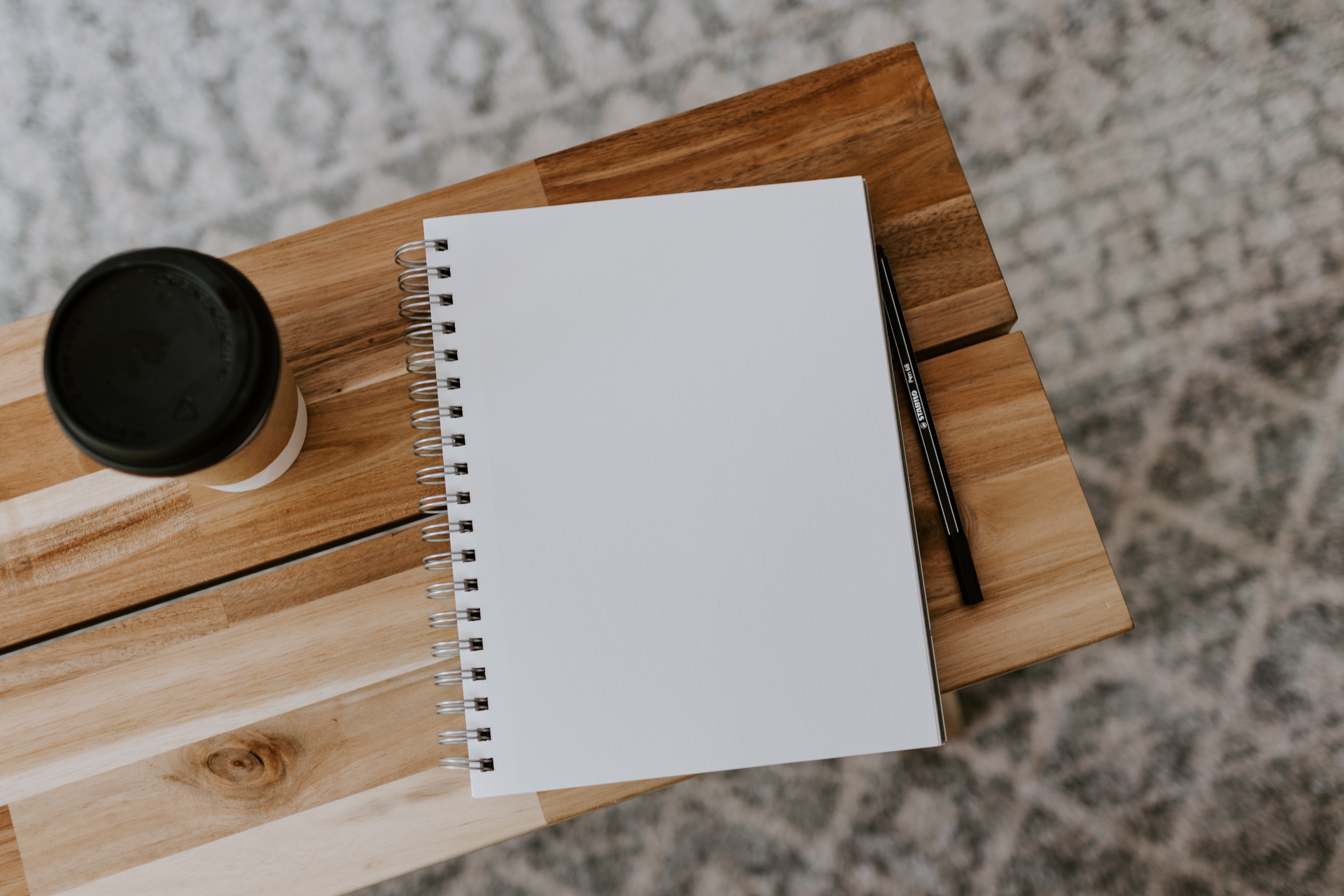
Survival Data Analysis
(MED1858) - Ευάγγελος Κριτσωτάκης, Χρήστος Θωμαδάκης
Περιγραφή Μαθήματος
MED1858 Survival Data Analysis
Evangelos Kritsotakis, Christos Thomadakis
Module summary
This module covers essential topics for analyzing time-to-event data or survival times. The emphasis is on practical aspects of applied data analysis in real-world datasets, without overlooking the underlying statistical theory. The module starts with illustrating the special features of survival data and introduces the basic mathematical functions central for describing and analysing survival times (the survival, hazard, and cumulative hazard functions), discussing their interrelationships and links to the cumulative distribution and probability density functions. A revision of basic theory in Maximum Likelihood Estimation and the Delta method for variance estimation takes place early on to ensure a smooth application of these methods in the context of survival analysis. We then explain methods for summarising survival data based on non-parametric techniques to estimate survival functions such as the Kaplan-Meier and the Nelson-Aalen estimators and consider the derivations of variances and confidence intervals. We proceed with methods for comparing survival time distributions between two or more groups and discuss the derivation of the Logrank test, its underlying assumptions, and its relation to other tests, such as the Wilcoxon test. The module then turns to regression models for survival outcomes, emphasizing on the formulation and application of the Cox proportional hazards model (estimation, testing, prediction and interpretation of model coefficients), and ways to assess the validity of the model in practice. Some more general issues in regression modelling are discussed, including model fit, assessment of linearity, flexible modelling, and variable selection. We then extend the Cox model to incorporate time-dependent explanatory variables. Alternative models based on the use of parametric distributions (exponential, Weibull) are also examined. Finally, we introduce methods for summarising times-to-event for different causes of event (competing risks), and describe models for cause-specific survival data. Class materials include lecture presentations and readings (journal papers and book chapters) along with Lab exercises and individual assignments using the R software for implementing survival analyses of real-world clinical and epidemiological studies. Stata code is also provided for optional study.
Recommended textbook
-
Collett: Modelling Survival Data in Medical Research (4th ed., 2023).
Other useful textbooks, available via Heal Link (access via university VPN):
- Kleinbaum (2012, 3rd ed). Survival Analysis: A self-learning text. HeaL-Link. Link 2. Link 3.
- Klein & Moeschberger (2003, 2nd ed.). Survival Analysis: Techniques for censored and truncated data. Heal-link.
- Liu (2012). Survival analysis: Models and applications. Heal-Link.
- Moore (2016). Applied Survival Analysis Using R. Heal-Link.
Timetable 2024
Unit |
Lectures (E. Kritsotakis) |
R Labs (C. Thomadakis) |
1. Introduction & basic functions in Survival Analysis |
02.10.2024, 4-6 pm ONL |
- |
2. Revision: Maximum Likelihood Estimation & Delta method |
03.10.2024, 4-6 pm ONL |
- |
3. Non-parametric estimation of the survival function |
14.10.2024, 4-6 pm |
14.10.2024, 6-8 pm |
4. Comparing survival functions |
16.10.2024, 4-6 pm |
16.10.2024, 6-8 pm |
5. Introduction to Cox regression |
18.10.2024, 4-6 pm |
18.10.2024, 6-8 pm |
6. More on Cox regression: Testing & predicting |
23.10.2024, 4-6 pm ONL |
23.10.2024, 6-8 pm ONL |
7. Cox regression: Linearity, Model Fit & Model Selection |
25.10.2024, 4-6 pm ONL |
25.10.2024, 6-8 pm ONL |
8. Assessing proportional hazards |
29.10.2024, 4-6 pm |
29.10.2024, 6-8 pm |
9. Time dependent variables |
31.10.2024, 4-6 pm |
31.10.2024, 6-8 pm |
10. Parametric Survival Analysis |
01.11.2024, 4-6 pm |
01.11.2024, 6-8 pm |
11. Competing Risks |
07.11.2024, 4-6 pm ONL |
07.11.2024, 6-8 pm ONL |
Assignments (individual submissions) |
Release date |
Deadline |
Homework 1 |
23.10.2024 |
11.11.2024 |
Homework 2 |
18.11.2024 |
20.12.2024 |
Ημερομηνία δημιουργίας
Πέμπτη 7 Οκτωβρίου 2021
-
Περιεχόμενο μαθήματος
This module covers essential topics for the analysis of time-to-event data or survival times. The emphasis is on practical aspects of applied data analysis in real-world datasets, without overlooking the underlying statistical theory. The module starts with introducing the basic mathematical functions that are central for describing and analysing survival times (the survival, hazard and cumulative hazard functions) and discusses their interrelationships as well as the links to the cumulative distribution and probability density functions. A revision of basic theory in Maximum Likelihood Estimation and the Delta method for variance estimation takes place early on to ensure a smooth application of these methods in the context of survival analysis. We then consider non-parametric techniques for estimating survival functions using the Kaplan-Meier and the Nelson-Aalen estimators, including derivation of their variances and calculation of confidence intervals. We proceed with methods for comparing survival time distributions and discuss the derivation of the Logrank test, its underlying assumptions and its relation to other tests, such as the Wilcoxon test. The module then turns to regression models for survival outcomes, emphasizing on aspects of the Cox proportional hazards model (estimation, testing, prediction and interpretation of model coefficients), and ways to assess the validity of the model in practice. Some more general issues in regression modelling are discussed, including the assessment of linearity, model fit and variable selection. We then extend the Cox model to incorporate time-dependent explanatory variables. Alternative models based on the use of parametric distributions (exponential, Weibull) are also examined. Finally, we review methods for summarising times-to-event for different causes of event (competing risks), and describe models for cause-specific survival data. Class materials include lecture presentations and readings (papers and book chapters) of statistical methods, along with Lab exercises and individual assignments using the R software for implementing survival data analyses of real-world clinical and epidemiological studies. Stata code is also provided for optional study.